概要
Git:Python的异步编程介绍(MD)。
博客
博客地址:IT老兵驿站。
前言
这里翻译和学习一篇介绍Python的异步编程的文章,在网上找了半天,感觉这篇写的很好,把几种实现方案都举了例子,而且列出了优劣。
之前的文章采用了富文本编辑,不太方便,这次改为MD格式。
正文
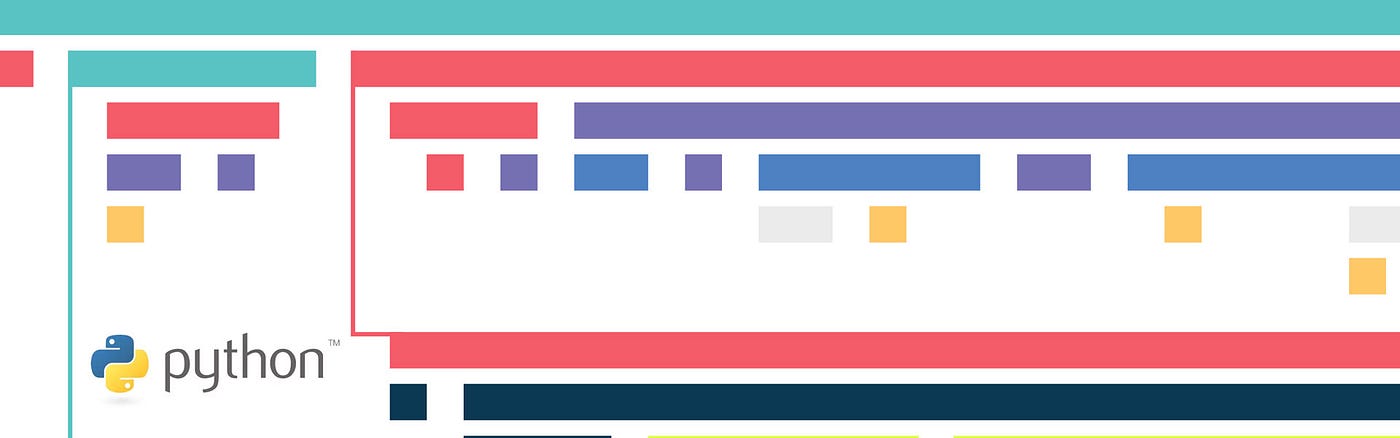
介绍 Introduction
Asynchronous programming is a type of parallel programming in which a unit of work is allowed to run separately from the primary application thread. When the work is complete, it notifies the main thread about completion or failure of the worker thread. There are numerous benefits to using it, such as improved application performance and enhanced responsiveness.
异步编程是一种并行编程,这里支持一个单元的工作被允许从主程序中分离出来。

Asynchronous programming has been gaining a lot of attention in the past few years, and for good reason. Although it can be more difficult than the traditional linear style, it is also much more efficient.
For example, instead of waiting for an HTTP request to finish before continuing execution, with Python async coroutines you can submit the request and do other work that’s waiting in a queue while waiting for the HTTP request to finish.
例如,与其等待一个 HTTP request 的返回,然后去继续后面的工作,就可以使用 Python async coroutines, 你提交了请求,然后就可以去做别的事情了,当请求回来的时候,它们会在一个队列里面等待。
Asynchronicity seems to be a big reason why Node.js so popular for server-side programming. Much of the code we write, especially in heavy IO applications like websites, depends on external resources. This could be anything from a remote database call to POSTing to a REST service. As soon as you ask for any of these resources, your code is waiting around with nothing to do. With asynchronous programming, you allow your code to handle other tasks while waiting for these other resources to respond.
异步性可能就是 Node.js 这么风靡的一个原因。
How Does Python Do Multiple Things At Once?
1. Multiple Processes 多进程方式
The most obvious way is to use multiple processes. From the terminal, you can start your script two, three, four…ten times and then all the scripts are going to run independently or at the same time. The operating system that’s underneath will take care of sharing your CPU resources among all those instances. Alternately you can use the multiprocessing library which supports spawning processes as shown in the example below.
多进程的实现方式是最常见的方式。从终端,你可以开启多份脚本去独立运行,这是一种方式;还有一种方式,就是语言层面的支持。
多进程实现的代码如下。
from multiprocessing import Process
def print_func(continent='Asia'):
print('The name of continent is : ', continent)
if __name__ == "__main__": # confirms that the code is under main function
names = ['America', 'Europe', 'Africa']
procs = []
proc = Process(target=print_func) # instantiating without any argument
procs.append(proc)
proc.start()
# instantiating process with arguments
for name in names:
# print(name)
proc = Process(target=print_func, args=(name,))
procs.append(proc)
proc.start()
# complete the processes
for proc in procs:
proc.join()
Output:
The name of continent is : Asia
The name of continent is : America
The name of continent is : Europe
The name of continent is : Africa
2. Multiple Threads 多线程方式
The next way to run multiple things at once is to use threads. A thread is a line of execution, pretty much like a process, but you can have multiple threads in the context of one process and they all share access to common resources. But because of this, it’s difficult to write a threading code. And again, the operating system is doing all the heavy lifting on sharing the CPU, but the global interpreter lock (GIL) allows only one thread to run Python code at a given time even when you have multiple threads running code. So, In CPython, the GIL prevents multi-core concurrency. Basically, you’re running in a single core even though you may have two or four or more.
多线程是在一个进程上下文中存在的,这样访问一些公共的资源是很容易的。但也同样是因为这一点,编写多线程代码是有些困难的。
多线程实现代码如下,这里有一个问题, GIL 是什么。
import threading
def print_cube(num):
"""
function to print cube of given num
"""
print("Cube: {}".format(num * num * num))
def print_square(num):
"""
function to print square of given num
"""
print("Square: {}".format(num * num))
if __name__ == "__main__":
# creating thread
t1 = threading.Thread(target=print_square, args=(10,))
t2 = threading.Thread(target=print_cube, args=(10,))
# starting thread 1
t1.start()
# starting thread 2
t2.start()
# wait until thread 1 is completely executed
t1.join()
# wait until thread 2 is completely executed
t2.join()
# both threads completely executed
print("Done!")
view rawgistfile1.txt hosted with ❤ by GitHub
Output :
Square: 100
Cube: 1000
Done!
3. Coroutines using yield 使用协程
Coroutines are generalization of subroutines. They are used for cooperative multitasking where a process voluntarily yield (give away) control periodically or when idle in order to enable multiple applications to be run simultaneously. Coroutines are similar to generators but with few extra methods and slight change in how we use yield statement. Generators produce data for iteration while coroutines can also consume data.
使用 yield 方式的协程。
def print_name(prefix):
print("Searching prefix:{}".format(prefix))
try :
while True:
# yeild used to create coroutine
name = (yield)
if prefix in name:
print(name)
except GeneratorExit:
print("Closing coroutine!!")
corou = print_name("Dear")
corou.__next__()
corou.send("James")
corou.send("Dear James")
corou.close()
Output :
Searching prefix:Dear
Dear James
Closing coroutine!!
4. Asynchronous Programming 异步编程
The fourth way is an asynchronous programming, where the OS is not participating. As far as OS is concerned you’re going to have one process and there’s going to be a single thread within that process, but you’ll be able to do multiple things at once. So, what’s the trick?
异步编程,这里 OS 并没有参与—-那应该只是语言自身的实现了。
使用asyncio的协程。
The answer is asyncio
asyncio is the new concurrency module introduced in Python 3.4. It is designed to use coroutines and futures to simplify asynchronous code and make it almost as readable as synchronous code as there are no callbacks.
asyncio uses different constructs: event loops, coroutines and futures.
- An event loop manages and distributes the execution of different tasks. It registers them and handles distributing the flow of control between them.
- Coroutines (covered above) are special functions that work similarly to Python generators, on await they release the flow of control back to the event loop. A coroutine needs to be scheduled to run on the event loop, once scheduled coroutines are wrapped in Tasks which is a type of Future.
- Futures represent the result of a task that may or may not have been executed. This result may be an exception.
上面这段是关键,asyncio使用了三个结构:event loops,coroutines 和 futures。
Using Asyncio, you can structure your code so subtasks are defined as coroutines and allows you to schedule them as you please, including simultaneously. Coroutines contain yield points where we define possible points where a context switch can happen if other tasks are pending, but will not if no other task is pending.
A context switch in asyncio represents the event loop yielding the flow of control from one coroutine to the next.
In the example, we run 3 async tasks that query Reddit separately, extract and print the JSON. We leverage aiohttp which is a http client library ensuring even the HTTP request runs asynchronously.
import signal
import sys
import asyncio
import aiohttp
import json
loop = asyncio.get_event_loop()
client = aiohttp.ClientSession(loop=loop)
async def get_json(client, url):
async with client.get(url) as response:
assert response.status == 200
return await response.read()
async def get_reddit_top(subreddit, client):
data1 = await get_json(client, 'https://www.reddit.com/r/' + subreddit + '/top.json?sort=top&t=day&limit=5')
j = json.loads(data1.decode('utf-8'))
for i in j['data']['children']:
score = i['data']['score']
title = i['data']['title']
link = i['data']['url']
print(str(score) + ': ' + title + ' (' + link + ')')
print('DONE:', subreddit + '\n')
def signal_handler(signal, frame):
loop.stop()
client.close()
sys.exit(0)
signal.signal(signal.SIGINT, signal_handler)
asyncio.ensure_future(get_reddit_top('python', client))
asyncio.ensure_future(get_reddit_top('programming', client))
asyncio.ensure_future(get_reddit_top('compsci', client))
loop.run_forever()
view rawgistfile1.txt hosted with ❤ by GitHub
Output :
50: Undershoot: Parsing theory in 1965 (http://jeffreykegler.github.io/Ocean-of-Awareness-blog/individual/2018/07/knuth_1965_2.html)
12: Question about best-prefix/failure function/primal match table in kmp algorithm (https://www.reddit.com/r/compsci/comments/8xd3m2/question_about_bestprefixfailure_functionprimal/)
1: Question regarding calculating the probability of failure of a RAID system (https://www.reddit.com/r/compsci/comments/8xbkk2/question_regarding_calculating_the_probability_of/)
DONE: compsci
336: /r/thanosdidnothingwrong -- banning people with python (https://clips.twitch.tv/AstutePluckyCocoaLitty)
175: PythonRobotics: Python sample codes for robotics algorithms (https://atsushisakai.github.io/PythonRobotics/)
23: Python and Flask Tutorial in VS Code (https://code.visualstudio.com/docs/python/tutorial-flask)
17: Started a new blog on Celery - what would you like to read about? (https://www.python-celery.com)
14: A Simple Anomaly Detection Algorithm in Python (https://medium.com/@mathmare_/pyng-a-simple-anomaly-detection-algorithm-2f355d7dc054)
DONE: python
1360: git bundle (https://dev.to/gabeguz/git-bundle-2l5o)
1191: Which hashing algorithm is best for uniqueness and speed? Ian Boyd's answer (top voted) is one of the best comments I've seen on Stackexchange. (https://softwareengineering.stackexchange.com/questions/49550/which-hashing-algorithm-is-best-for-uniqueness-and-speed)
430: ARM launches “Facts” campaign against RISC-V (https://riscv-basics.com/)
244: Choice of search engine on Android nuked by “Anonymous Coward” (2009) (https://android.googlesource.com/platform/packages/apps/GlobalSearch/+/592150ac00086400415afe936d96f04d3be3ba0c)
209: Exploiting freely accessible WhatsApp data or “Why does WhatsApp web know my phone’s battery level?” (https://medium.com/@juan_cortes/exploiting-freely-accessible-whatsapp-data-or-why-does-whatsapp-know-my-battery-level-ddac224041b4)
DONE: programming
view rawgistfile1.txt hosted with ❤ by GitHub
上面这段代码有点过时了,现在已经不是太推荐这种用法了,不再直接对 loop 进行操作,而是对 task 进行处理,参考官网,但是这种用法可能是最常见的一种用法。
Using Redis and Redis Queue RQ
老版本Python的办法,不支持 asyncio 和 aiohttp 的版本怎么办,使用Redis 和 RQ。下面的文章意义已经不大了,就不过多记录了。
Using asyncio and aiohttp may not always be in an option especially if you are using older versions of python. Also, there will be scenarios when you would want to distribute your tasks across different servers. In that case we can leverage RQ (Redis Queue). It is a simple Python library for queueing jobs and processing them in the background with workers. It is backed by Redis — a key/value data store.
In the example below, we have queued a simple function count_words_at_url using redis.
from mymodule import count_words_at_url
from redis import Redis
from rq import Queue
q = Queue(connection=Redis())
job = q.enqueue(count_words_at_url, 'http://nvie.com')
******mymodule.py******
import requests
def count_words_at_url(url):
"""Just an example function that's called async."""
resp = requests.get(url)
print( len(resp.text.split()))
return( len(resp.text.split()))
view rawgistfile1.txt hosted with ❤ by GitHub
Output:
15:10:45 RQ worker 'rq:worker:EMPID18030.9865' started, version 0.11.0
15:10:45 *** Listening on default...
15:10:45 Cleaning registries for queue: default
15:10:50 default: mymodule.count_words_at_url('http://nvie.com') (a2b7451e-731f-4f31-9232-2b7e3549051f)
322
15:10:51 default: Job OK (a2b7451e-731f-4f31-9232-2b7e3549051f)
15:10:51 Result is kept for 500 seconds
view rawgistfile1.txt hosted with ❤ by GitHub
Conclusion 结论
Let’s take a classical example, a chess exhibition where one of the best chess players competes against a lot of people. And if there are 24 games with 24 people to play with and the chess master plays with all of them synchronically, it’ll take at least 12 hours (taking into account that the average game takes 30 moves, the chess master thinks for 5 seconds to come up with a move and the opponent — for approximately 55 seconds). But using the asynchronous mode gives chess master the opportunity to make a move and leave the opponent thinking while going to the next one and making a move there. This way a move on all 24 games can be done in 2 minutes and all of them can be won in just one hour.
So, this is what’s meant when people talk about asynchronous being really fast. It’s this kind of fast. Chess master doesn’t play chess faster, the time is just more optimized and it’s not get wasted on waiting around. This is how it works.
In this analogy, the chess master will be our CPU and the idea is that we wanna make sure that the CPU doesn’t wait or waits the least amount of time possible. It’s about always finding something to do.
A practical definition of Async is that it’s a style of concurrent programming in which tasks release the CPU during waiting periods, so that other tasks can use it. In Python there are several ways to achieve concurrency , based on our requirement, code flow, data manipulation , architecture design and use cases we can select any of these methods.
参考
href=”https://medium.com/velotio-perspectives/an-introduction-to-asynchronous-programming-in-python-af0189a88bbb“ rel=”nofollow”>https://medium.com/velotio-perspectives/an-introduction-to-asynchronous-programming-in-python-af0189a88bbb
https://docs.python.org/3/library/asyncio-task.html